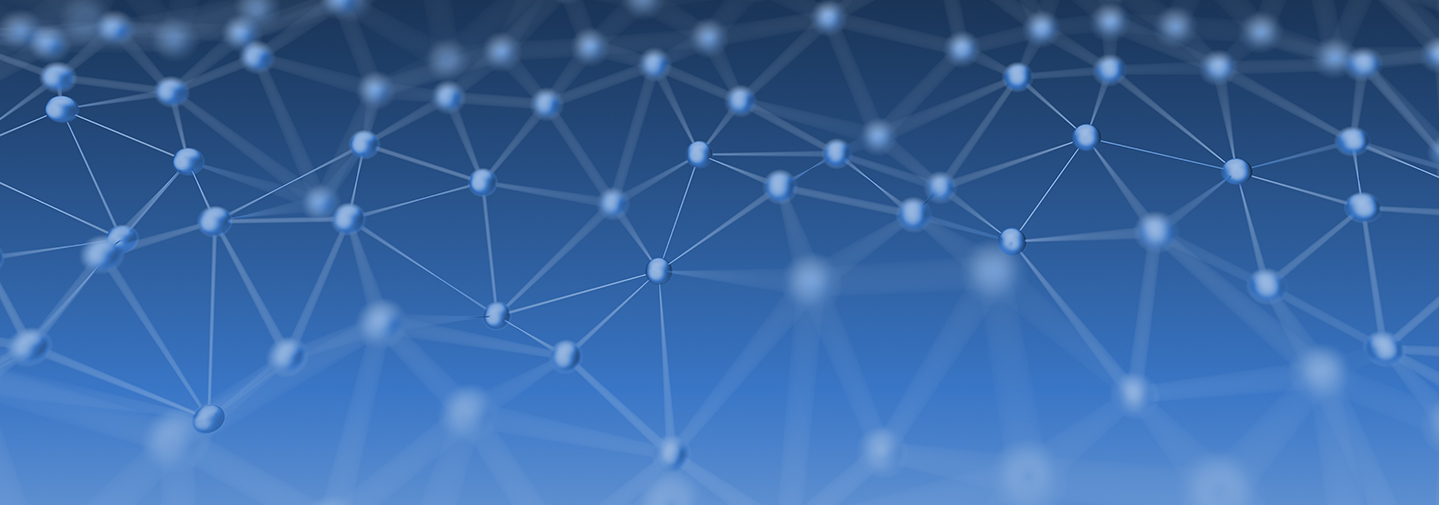
Today companies are facing big challenges dealing with Cyber Security. They are losing money, brand reputation and trust because of Cyber attacks. These attacks come from external hackers as well as insiders. Insider threats are hardest to catch because
employees are given access and permissions and its very hard to differentiate between normal activity vs rogue activity.
To detect rouge insiders or external hackers with stolen credentials as soon as possible companies need to go beyond security event analysis (SIEM). They need to track user/employee behavior to detect threats/anomalies.
User behavior analytics (UBA) helps in creation of user baseline profile and tracking deviations from normal behavior so companies can quickly detect insider threats.
UBA is part of InsightLake Security Intelligence Solution. Security Intelligence solution allows companies to collect different types of events/flows from network assets and external threat feeds easily at central place, apply co-relations and threat models to detect various attacks. InsightLake UBA solution applies machine learning algorithms to detect anomalies, comparing/baselining users with their peer groups and continuous profiling of users. Solution enables security admins to see users, their roles, hierarchies, activities, risk categories and co-related security events. It allows them to create watch lists of users and drill down in flows to identify threats.
InsightLake UBA detects various types of insider threats:
Type of suspicious activities
InsightLake data management solution allows collection of user actions/events data from various system logs and agents in real time using an intuitive UI. To build a robust and effective user baseline we need to collect all user interactions with enterprise assets.
Users typically access data, applications and network assets in a defined way. For example an employee would work during normal work hours, login to systems, access files & databases, print documents, send emails etc. They either use workplace or VPN to connect to network assets. When they use VPN they might use it from limited number of locations. Users will typically access limited set of data stores based on their granted permissions. They will query/download data in some size band. Typically users in a given business unit, having same roles and job functions would access data similarly. Some times there is a normal need to work off hours, get urgent privileged access, operate on data to fix or perform something. Capturing this exception early in user profile will reduce false positives. It is important that we capture exceptional requirements through security channel and utilize user's role and granted permissions so user profile reflects that. Once we build a user profile we baseline different patterns. Any time there is a deviation from set or known normal pattern we need to raise a critical event so anomalous behavior can be inspected. InsightLake allows analyst to enrich user profiles and set thresholds for "user to asset" interaction. Users baselines are regularly updated and any anomalies are checked by taking seasonality into account as well using unsupervised machine learning algorithms.
Risk scores are calculated for each user and updated regularly. Risk score contains following weighted parameters:
Once security analyst identifies threat users they can find out other users who are exhibiting similar patterns. They can find out insider threat rings.
Security admins can put users in watch lists and manage them through interactive UI.
UBA solution continuously profiles users/entities on a rolling window and creates risk scores which are recent and more relevant.
UBA solution uses entity centric event modeling to create a robust homogenized data set for users/entities (service accounts). This data set enables complex machine learning models to perform better (higher precision prediction).
InsightLake UBA solution utilizes many machine learning models to segment/classify users, detect patterns and anomalies. Both supervised and un-supervised algorithms are used along with security admin rules. Deep learning is used in models to detect users early on in their access patterns to contain the damage.
InsightLake UBA dashboard provides a single view of all enterprise users with following dashboards: